Who we are
- Every day, we work to create a desirable future.
- We've been around for over 50 years.
- We file over 600 patents a year.
- We form a group comprising four institutes (List, Leti, Liten, CEA Reg)
- We are tackling 19 priority challenges for society through our 54 world-class technology platforms.
- We are a multidisciplinary team of over 4,000 people with scientific, technical and administrative backgrounds.
Are you ready to become a citizen with a job that makes an impact?
Are you ready to join an organization and be of service to business, society and the planet?
We have the power to act, and we suggest you use it!
CEA-DRT at a glance


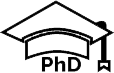


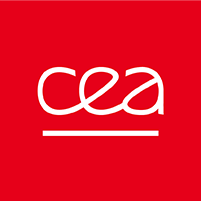
Our latest job opportunities
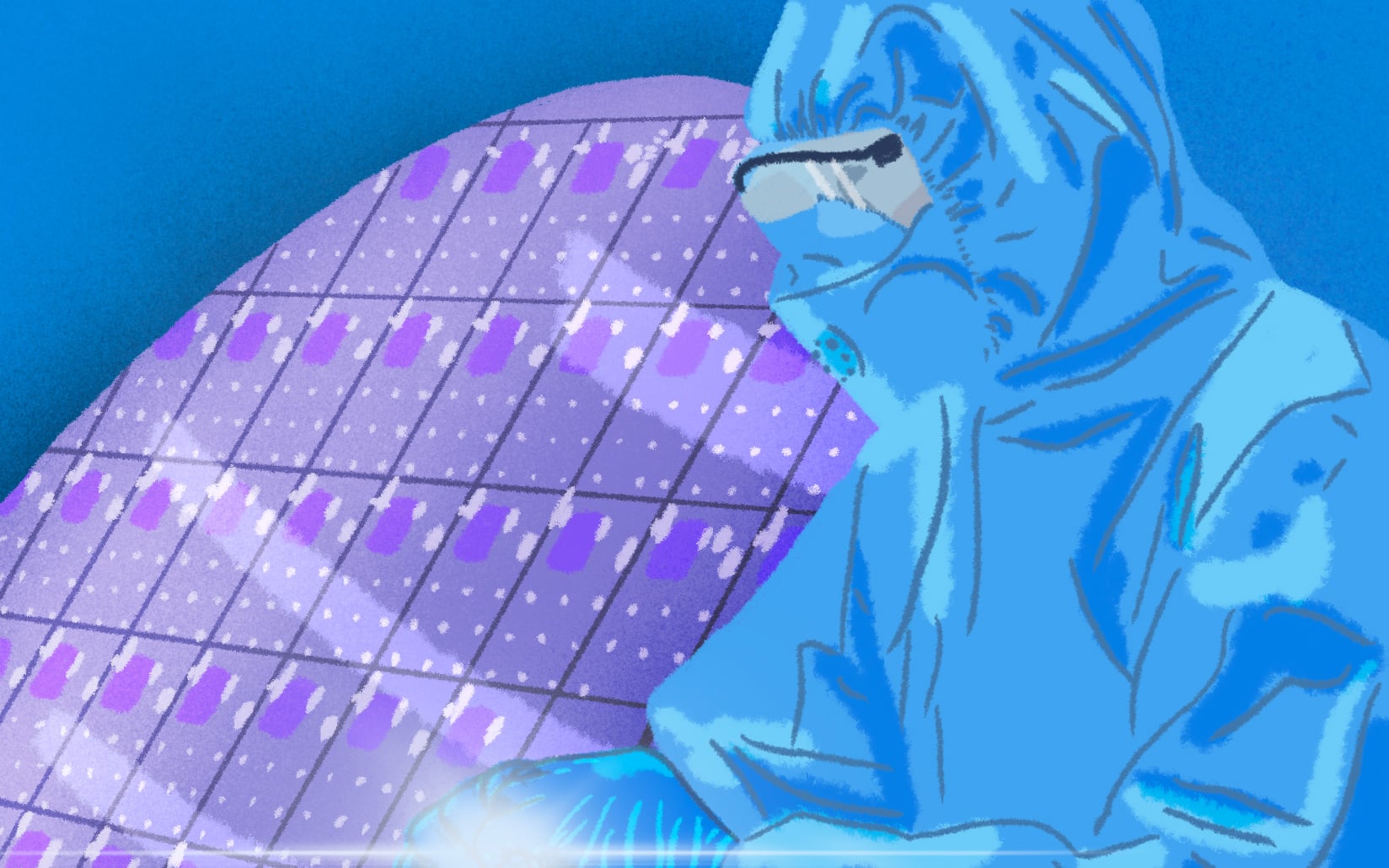
- Fixed term contract
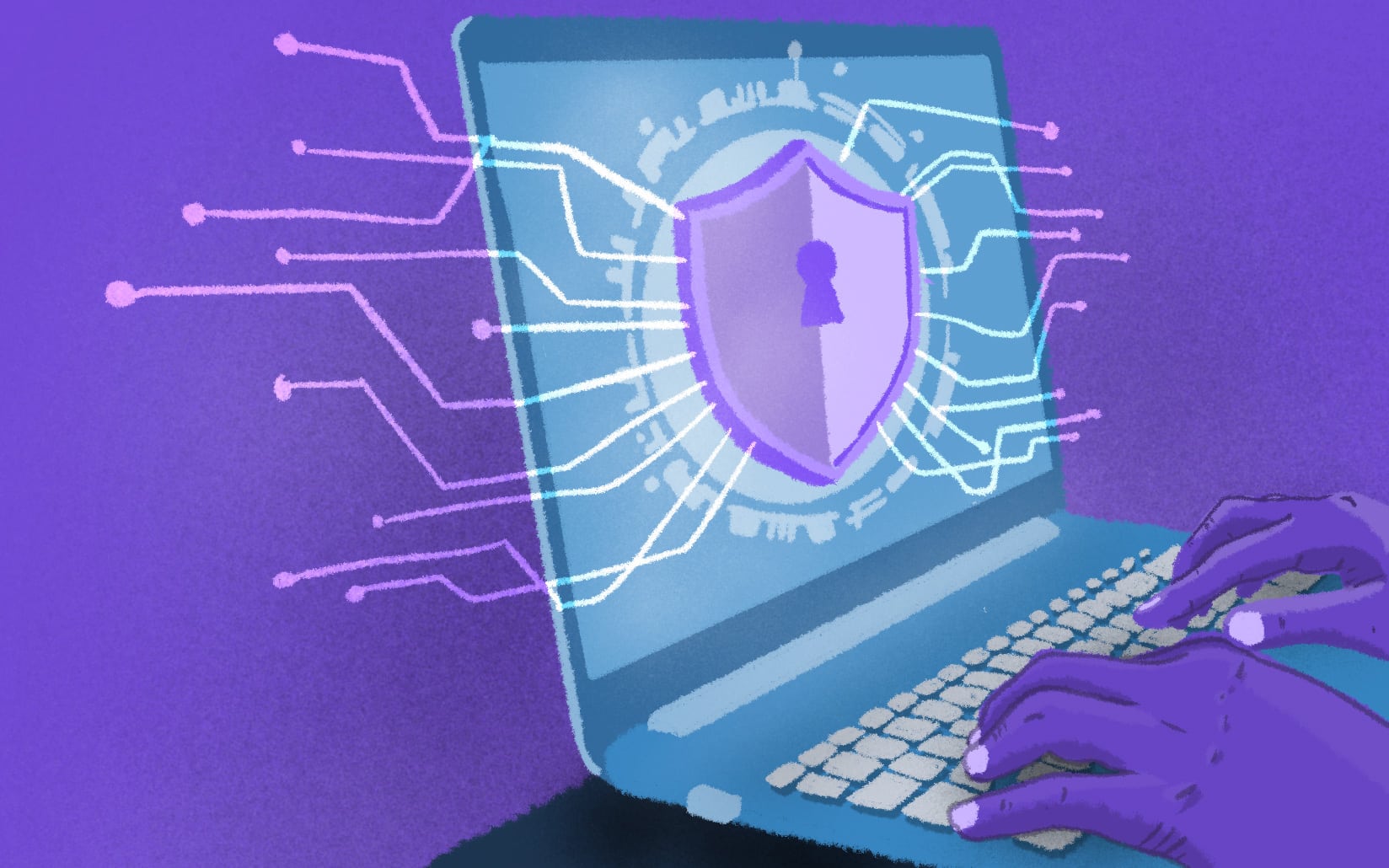
- Fixed term contract
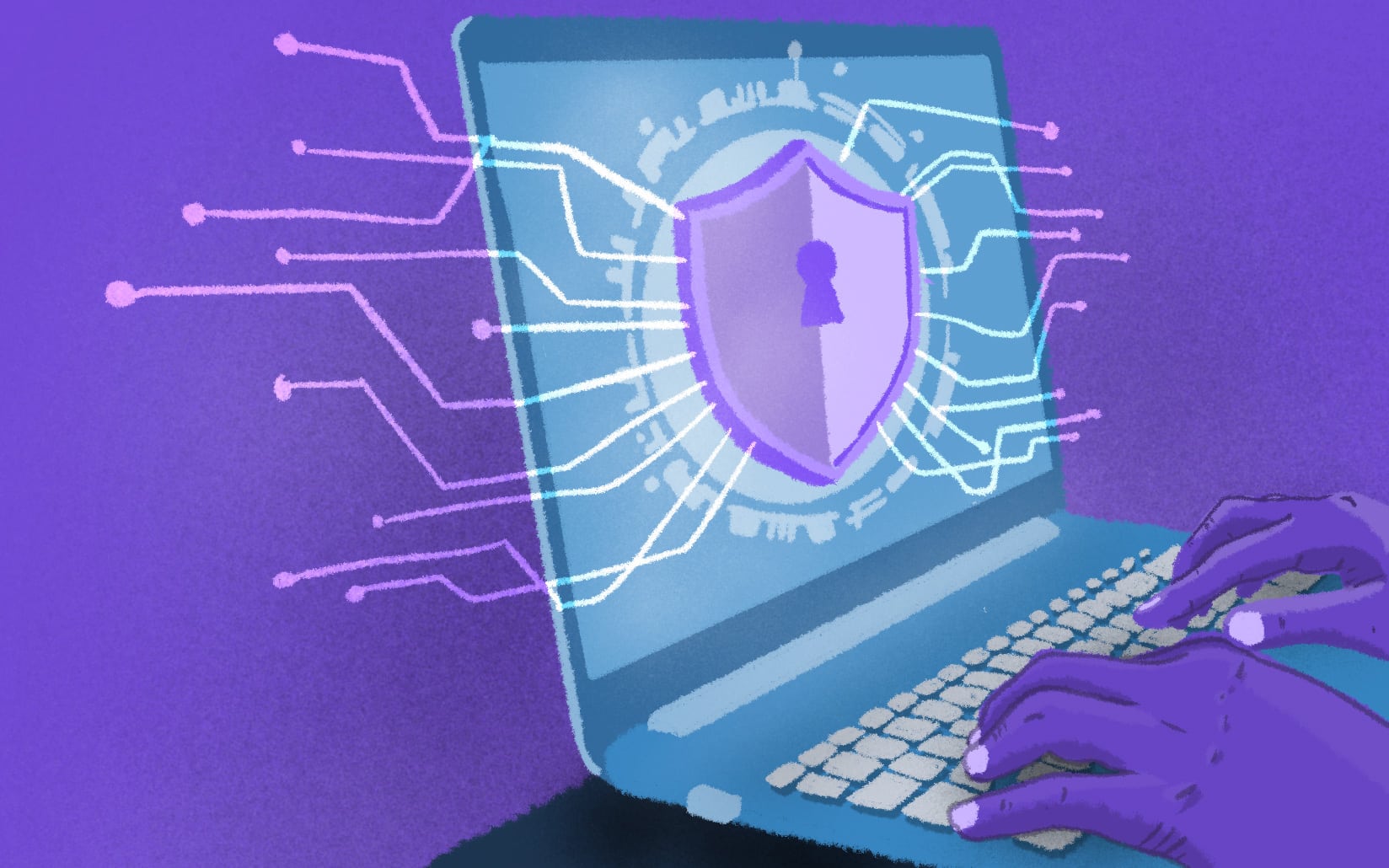
- Fixed term contract
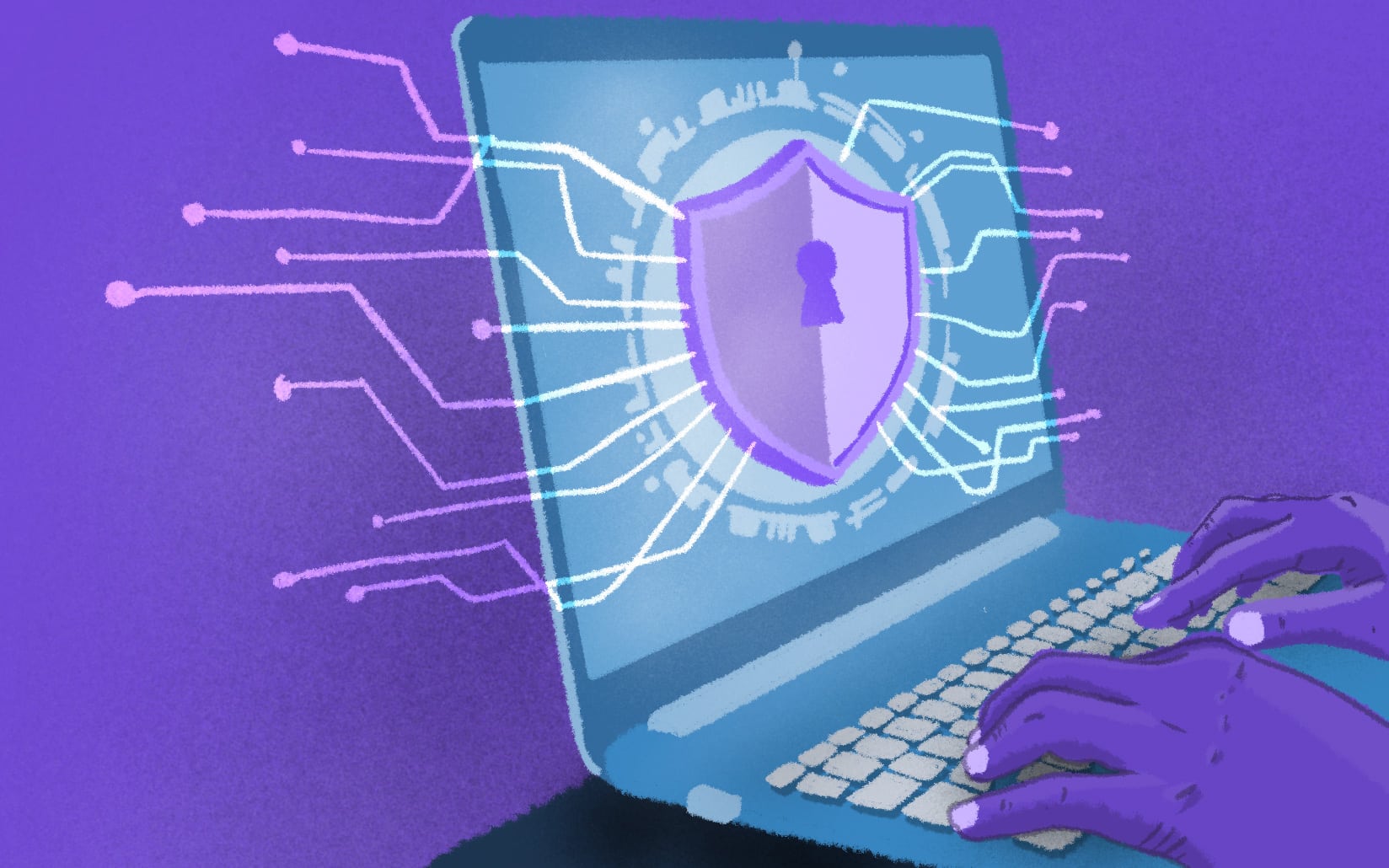
- Permanent contract
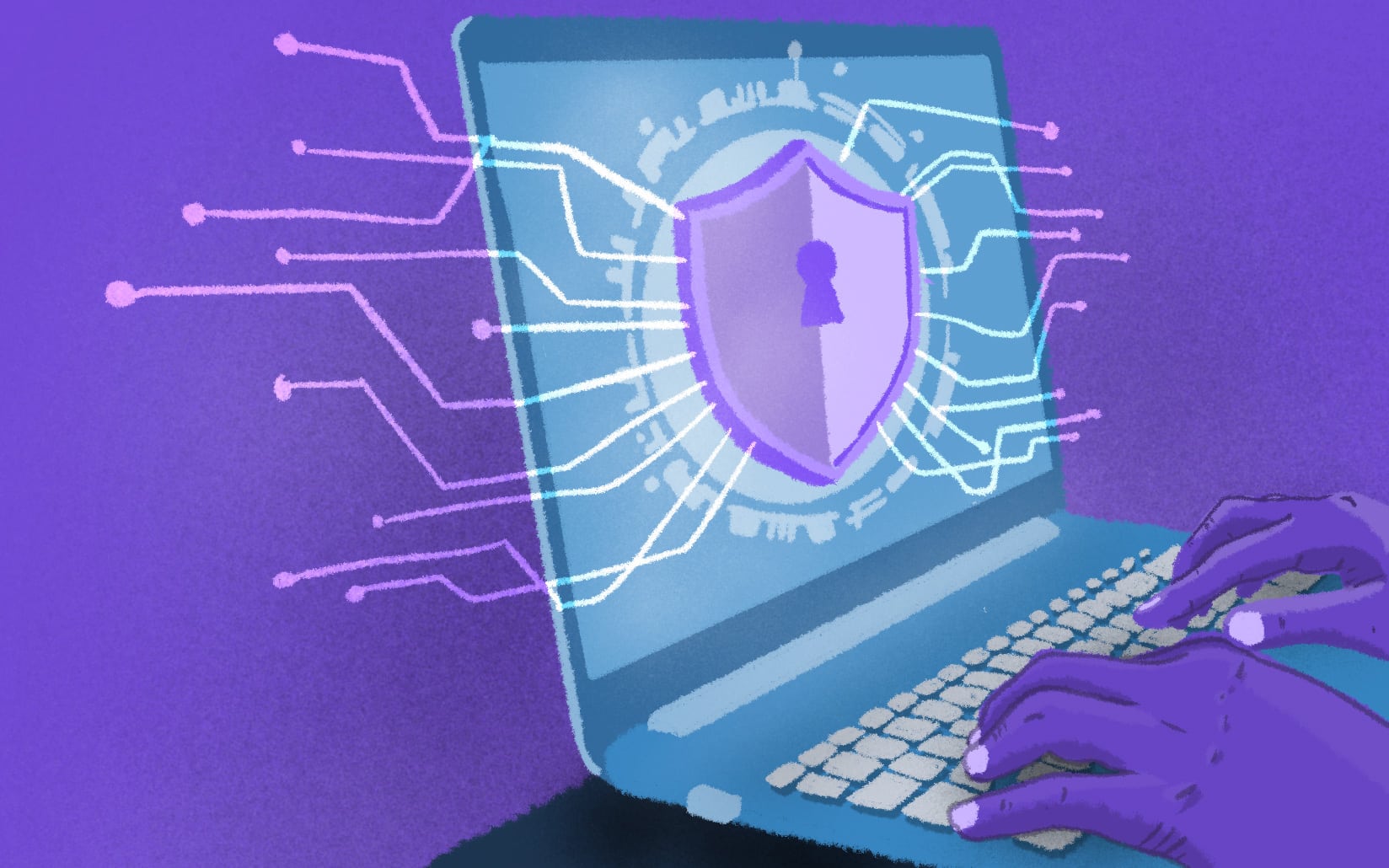
- Permanent contract
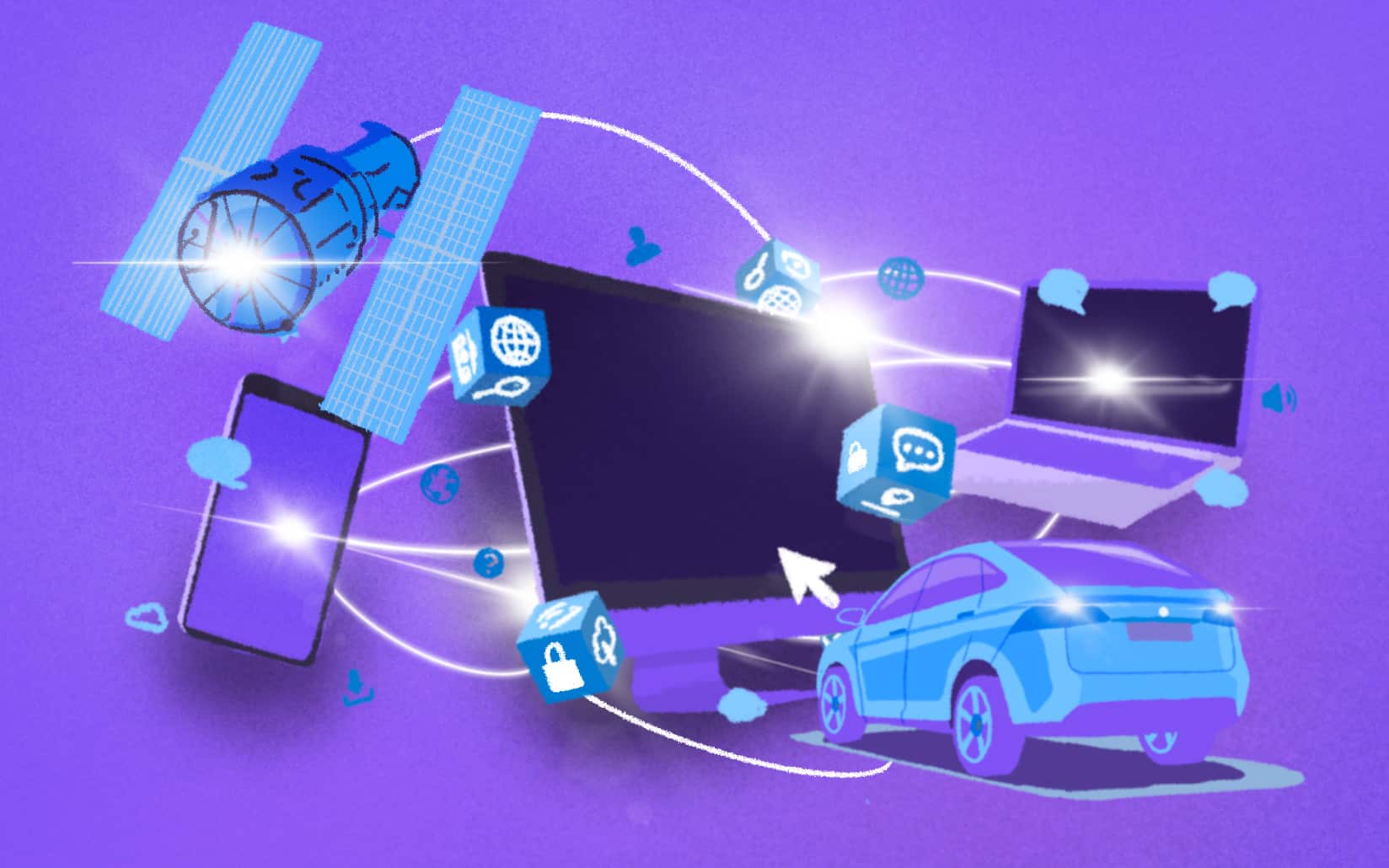
- Fixed term contract
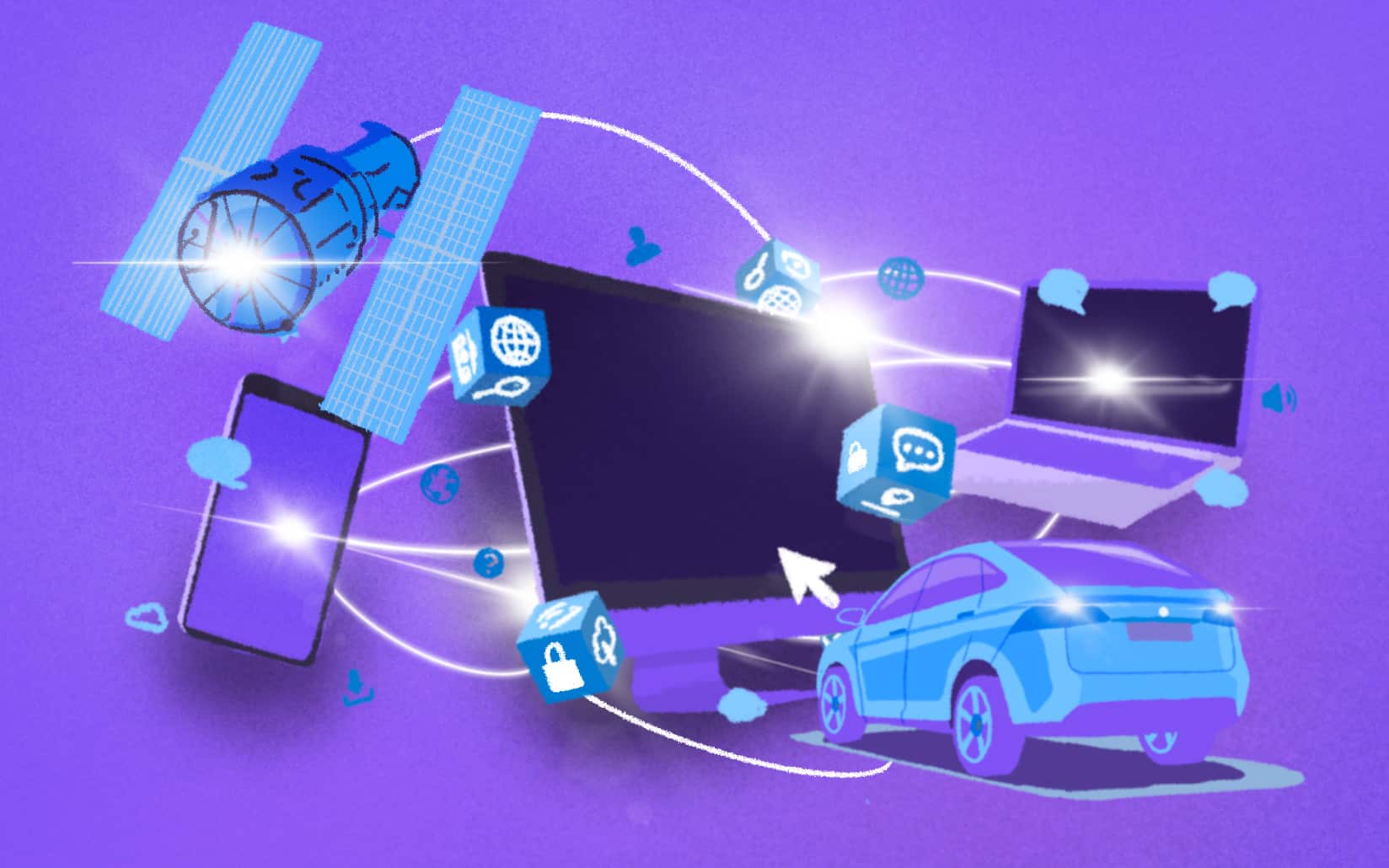
- Permanent contract
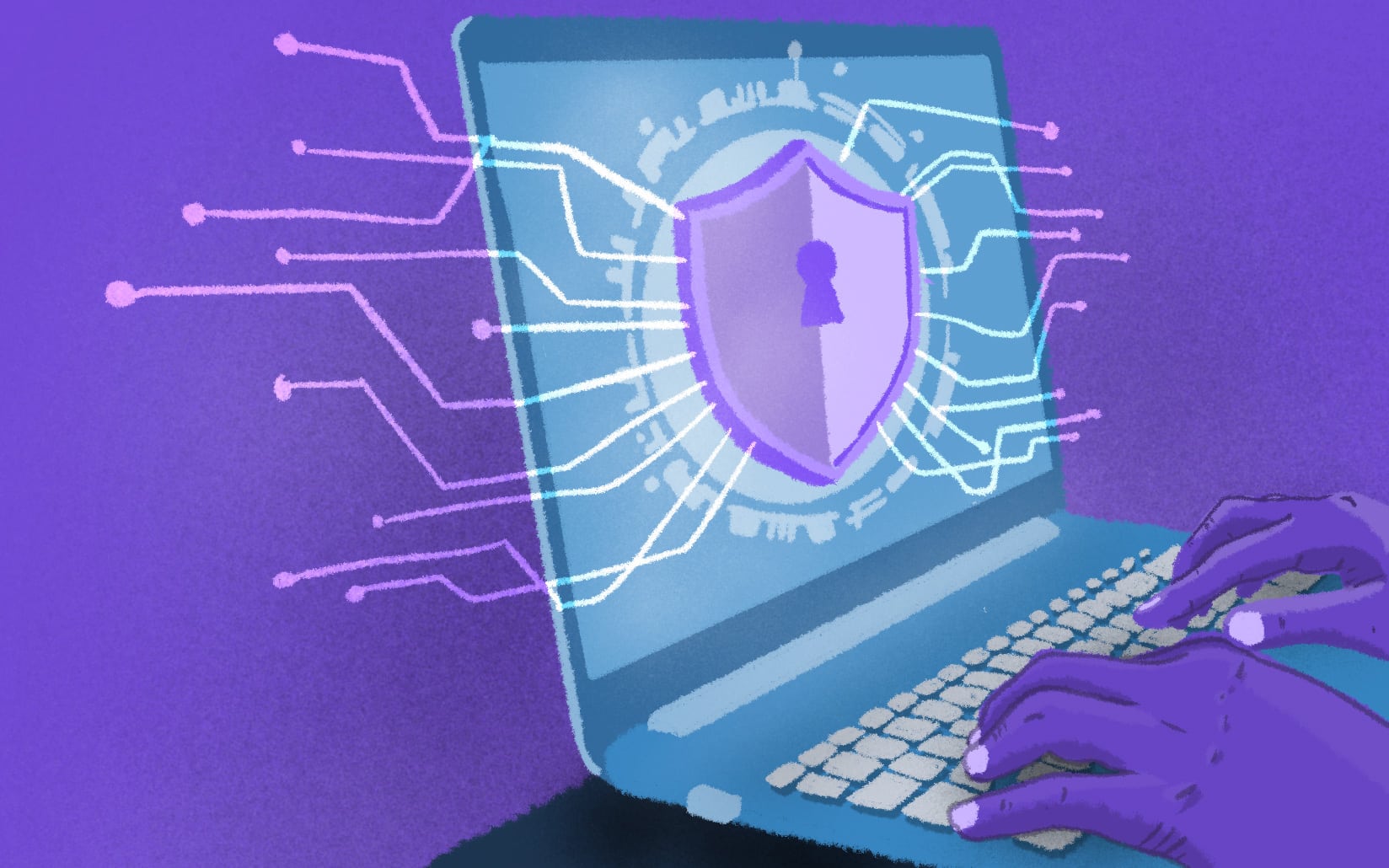
- Fixed term contract
Why join us
Joining the CEA's DRT means joining a close-knit team of 4,000 researchers, engineers and technicians based at our historic sites in Paris-Saclay and Grenoble, or in the regions.
It means being accompanied and supported by recognized experts in their fields. It means having the keys to developing your skills.
Joining our research teams means working in the best French and European innovation ecosystems. It means working with the region's most innovative players to test new ideas and push back the boundaries.
Que votre métier soit scientifique, technique ou administratif, rejoindre la DRT du CEA c’est contribuer à construire un futur plus désirable en :
- Developing hardware and software solutions for a frugal and responsible digital world
- Promoting the emergence of low-carbon energy and reducing resource consumption
- Imagining the medicine of the future, more personalized, more humane and more effective
- Guaranteeing France's technological and industrial sovereignty and strategic autonomy.
thanks to a concentration of expertise, software resources and cutting-edge hardware that is unique in Europe.
Avec plus de 50 plateformes technologiques au niveau des plus hauts standards industriels et une concentration d’expertises et de moyens unique en Europe, nos équipes développent dans des délais optimisés, des produits innovants, protégés par une propriété intellectuelle forte.
With a portfolio of 6,500 active patent families, DRT is a major player in technological research in France, Europe and the rest of the world.
Every year, our proactive intellectual property policy leads us to register over 600 new priority patents.
Becoming PhD student
at CEA
Becoming a PhD student at CEA is an exceptional opportunity for students who wish to pursue a career as a researcher.
Being a PhD student at CEA means working on innovative research projects, in response to technological challenges facing industry and society, for a desirable future. défis technologiques industry and society, for a desirable future.
Being a PhD student at CEA means benefiting from high-level scientific training, personalized supervision, and access to world-class research facilities and equipment.
Guided by renowned experts, our PhD students evolve in a collaborative and stimulating work environment, where they can develop their skills and make proposals to advance science.
Being a PhD student at CEA is an opportunity to develop your network through close collaborations with CEA's industrial and academic partners.
Become a PhD student.
This is your time to take your next step at the CEA !
Our technology challenges
News
All news
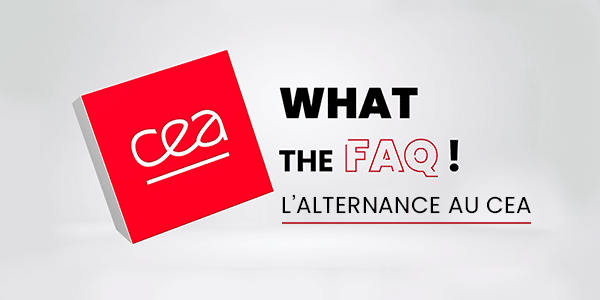
The Technology Research Division's work-study recruitment campaign is underway! Every year, we recruit over 150 work-study students.
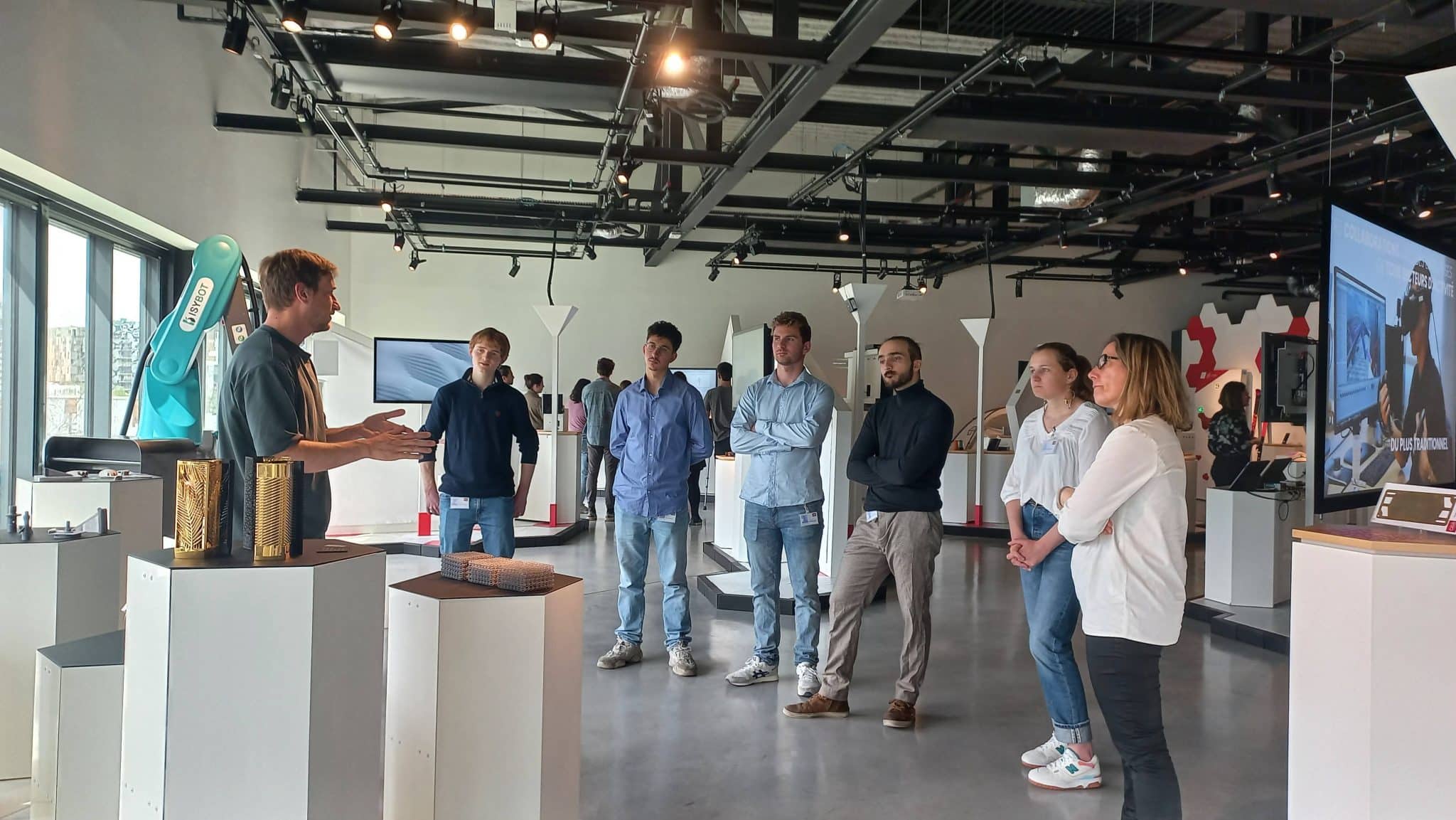
Grenoble, April 4, 2024 - The CEA's Technological Research Division (DRT) welcomed ENSE3 students for an immersive day, revealing the [...].
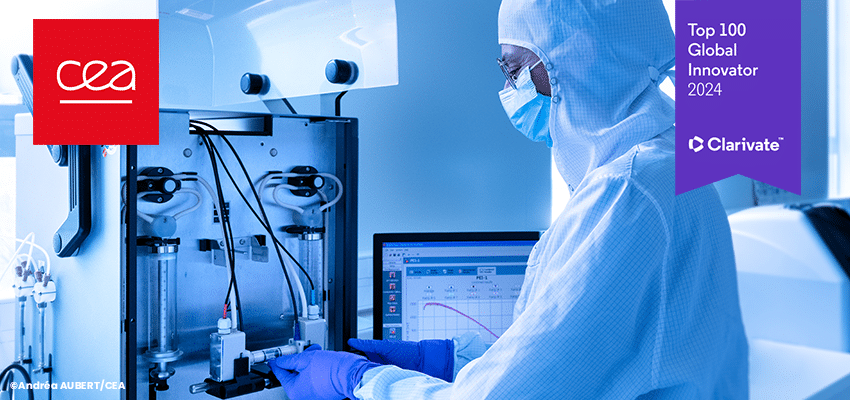
The CEA has once again distinguished itself in Clarivate's TOP 100 Global Innovators 2024, ranking 31st and marking its 12th [...].